
- Created2021.06.09
Medical Imaging Diagnostic Equipment Becomes Smarter Through Big Data and Deep Learning
-KIMM adds machine learning to diagnostic ultrasound equipment -
- Benefits of both improved diagnosis speed and accuracy -
The Korea Institute of Machinery and Materials (KIMM, President Sang-Jin Park), an institution under the jurisdiction of the Ministry of Science and ICT, has developed technology that improves both the speed and accuracy of disease diagnosis by combining medical imaging diagnostic equipment with the machine learning technology of mechanical equipment.
Led by Dr. Jong-won Park, head of the Department of Reliability Assessment at KIMM, the research team applied big data deep learning technology that has been used to diagnose the reliability of mechanical parts and equipment to ultrasound imaging equipment to develop diagnostic imaging assistance technology using machine learning. Such technology utilizes a GPU to achieve a required diagnosis time of 30 minutes, with an accuracy of 80%.
Recently in the medical field, ultrasound imaging, computed tomography, magnetic resonance imaging, and other forms of diagnostic image-based machine learning technologies are being actively utilized in the early diagnosis of heart and brain diseases.
In looking for ways to combine machine learning techniques used for mechanical parts and equipment reliability inspection with aspects of the medical field, the research team cooperated with the cardiology research team at Daejeon St. Mary’s Hospital. Together, they conducted academic research using artificial intelligence deep learning to develop a cardiovascular event prediction model through automated analysis of aortic atherosclerotic plaque found in patients suffering from cerebral infarction. The study was successful in confirming the effectiveness of such methods.
There have been several attempts to apply machine learning to the medical field, but this particular study was a new approach toward developing a deep learning model that can be used to classify aortic plaque and measure plaque thickness.
The research team applied the autoencoder and U-Net models, standard machine learning techniques, in differentiating between the ultrasound images of the aortic wall. By identifying the aortic wall using ultrasound images, the conditions of any aortic atherosclerotic plaque, which are now considered to play a role in the onset of stroke, can be confirmed.
Dr. Jong-won Park, head of the Department of Reliability Assessment at KIMM, stated that, until now, users were required to have complex data analysis skills to determine the failure or lifespan of mechanical parts and equipment, but now they can easily access such information through various open sources. He added that imaging technology is expected to be implemented across various fields in the future, such as in the detection of various diseases and in the development of prediction models for the reliability of various parts and equipment.
The research team plans to modify the deep learning model to improve the accuracy of aortic plaque analysis. In the future, the team also plans to expand upon this technology, so that it that can be used along with imaging data for diagnosing faults and failures in the establishment of virtual engineering platforms for manufacturing future transportation equipment parts and in projects operated by the Materials and Parts Convergence Alliance in the mechanical and automobile sectors.
[List of Attachments] - Attachment 1: Glossary of Key Terms
- Attachment 2: Structure and Results of the Autoencoder Model (Image)
- Attachment 3: Structure and Results of the U-net Model (Image)
The Korea Institute of Machinery and Materials (KIMM) is a non-profit governmentfunded research institute under the Ministry of Science and ICT. Since its foundation in 1976, KIMM is contributing to economic growth of the nation by performing R&D on key technologies in machinery and materials, conducting reliability test evaluation, and commercializing the developed products and technologies.
This research project was conducted with support from the Ministry of Trade, Industry and Energy and the project for the establishment of virtual engineering platforms for manufacturing future transportation equipment parts, which is part of the program for technology-based innovation for materials and parts.
Credit : The Korea Institute of Machinery and Materials (KIMM)
Usage Restrictions of Multimedia (Attachment File) : The sources of photos and research results from KIMM must be specified.
- Attachment 1: Glossary of Key Terms
1. Reliability Reliability describes the characteristic of a given item, such as unit, part, product, or system, to perform a given function without failure for a given period of time under given conditions of use. When this characteristic is expressed as a quantitative measurement of probability, it is referred to as degree of reliability.
2. Machine Learning Machine learning is a field of artificial intelligence in which technologies and techniques are implemented using computers to achieve various functions, such as the learning ability of human beings.
3. Deep Learning Deep learning is a type of machine learning technology based on artificial neural networks. This technology enables computers to recognize, reason, and make judgments all on its own, in a similar fashion to how a huge network of neurons or nerve cells is activated when a person receives certain information to recognize things or make judgments.
4. Autoencoder Autoencoder is a deep learning method that uses input and output layers that are composed symmetrically on the left and right. Learning is conducted through the extraction of specific features by compressing the original data through encoders and restoring that information through decoders.
5. U-net RU-net is a deep learning method that is characterized by its U-shape when structurally expressed as an artificial neural network for image segmentation. Starting from the center, the encoder structure where the image is reduced is on the left, and the decoder structure where the image is enlarged to its original size is on the right. One of the advantages to this method is that it is relatively fast, for the image is newly verified from the next area, without the need for duplicate verification in any overlapping areas.
6. Plaque In the blood vessels, plaque is formed due to the build-up of foreign substances that stick to each other and to the walls of the blood vessels. When plaque is formed, it combines with other substances, such as calcium, to form a hard exterior, thus gradually causing the blood vessels to become narrower and potentially leading to arteriosclerosis.
- Attachment 2: Structure and Results of the Autoencoder Model (Image)
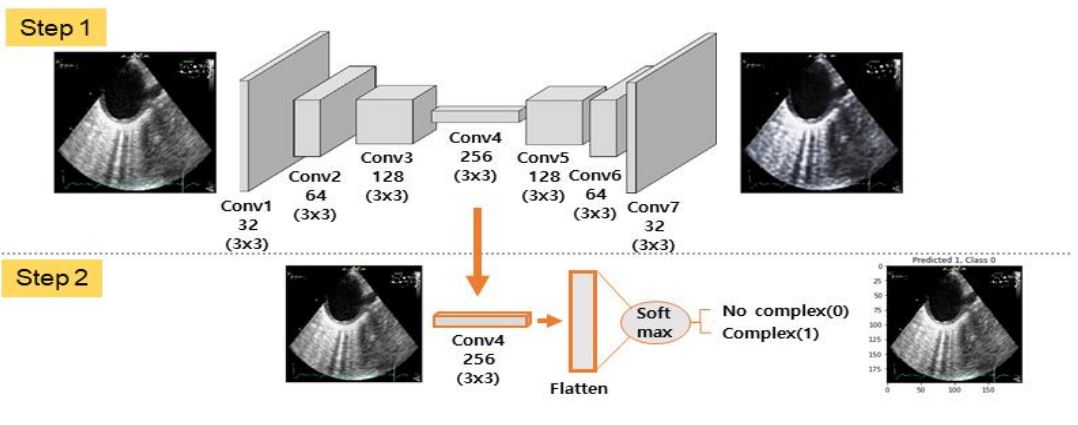
Image description: Plaque classification using the Autoencoder deep learning model
This process utilizes the autoencoder model in two steps to classify the condition of plaque through ultrasound imaging. Step 1 is a learning process in which the original image is inputted and seven convolution layers are created to create an output image that is similar to the original input. In step 2, the fourth convolution layer, in which the weighted values learned in step 1 are saved, are applied to create a fully connected layer, after which the plaque condition values are set to either normal (No complex and coded as 0) or complex (Complex and coded as 1) using the softmax function. The results learned through the autoencoder model are expressed as the plaque condition values as predicted by the deep learning model like the image from the last stage of step 2, Predicted and 0 or 1, and the plaque condition values as determined by a physician, Class and 0 or 1.
- Attachment 3: Structure and Results of the U-net Model (Image)
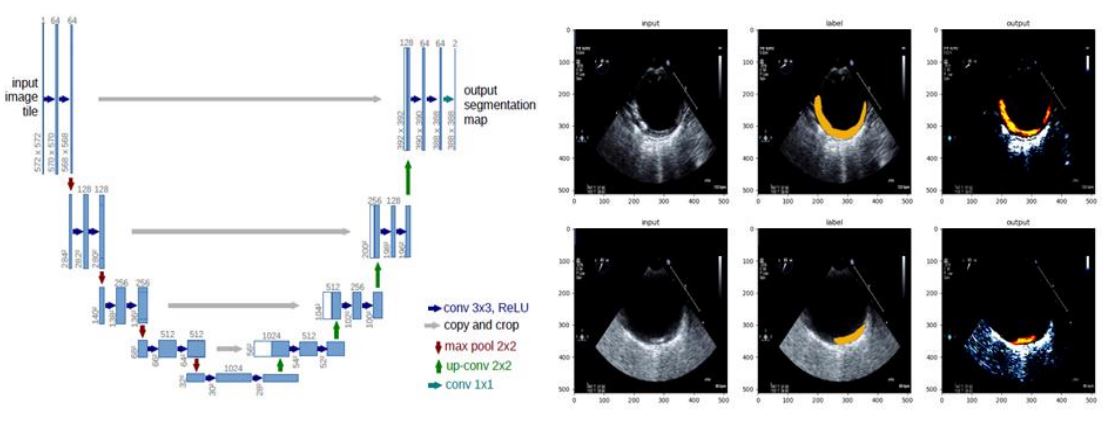
IImage description: Plaque area prediction using the U-net deep learning model
(Left) On the left, the deep learning structure of the U-net model is composed of a symmetrical structure with two sub-networks: the contracting path for contracting a given image, and the expanding path for expanding a given image size using the feature map obtained from the contracting path. This particular model can maintain and minimize the loss of any given information by combining the existing information without discarding it as the input image goes through the U-shaped process of contraction and expansion.
(Right) As shown in the middle image on the right-hand side, labeled images are created with plaque areas labeled in orange, based on where the plaque measurement values are marked on the image data. When the first test image is inputted by learning the labeled image from the original image, the labeled image, which displays the predicted plaque areas, is shown as the result of the last column.