Algorithm that analyzes the signal measured from main
components of the wind turbine to analyze/assess their health in
real-time and provides information required for operation and
maintenance(O&M).
It is based on the condition monitoring system (CMS) for real-time
diagnosis of the wind turbine system. While conventional CMSs
only detect existences of the fault, this technology provides the fault
diagnosis of main components and also predicts the remaining
useful life(RUL) of the components.
For the fault diagnosis and prediction of RUL in this technology,
big data processing and AI/machine learning techniques (neural
network, extended hidden Markov model) are applied to improve the
accuracy of fault diagnosis to 99%, and with the statistical inferences
including the Bayesian method and Monte Carlo simulation, the
error in estimated RUL was reduced to 10% or lower.
The fault diagnosis and RUL prediction for main components can be
effectively continued during the varying load due to the natural wind.
Client / Market
- Wind turbine, power plant, chemical plant, aircraft and railroad vehicle using power
train system
Necessity of this Technology
- To consider economic aspects, a large capacity wind power generation system is
built in a large complex and increase of the length of the blades for higher output
leads to increase in the load working on the tower and the blades itself, which
increases the risk of wind power generation system damage. Therefore, the
developments of diagnosis and maintenance technology to reduce breakdown time
and increase availability are critical to guarantee profitability of the plants.
- There is a need in offshore wind farm for technology development to monitor, diagnose
and predict the conditions of main components including blades, gearbox, and the
generator considering SCADA simultaneously in order to maximize availability.
- More than 40% of the faults in wind turbines installed in Europe in the past 20 years
were caused by faults of machines and components. But majority of wind turbines
installed in Korea are experiencing a serious difficulty with O&M due to contractrelated
or technical issues, and this is the results of inexperienced operation
techniques and insufficient condition monitoring technologies.
- Conventional CMS only detects whether the measured signal exceeds the normal
value or whether a fault has occurred. Therefore, prediction technology for faults is
necessary to enable active risk management of the target system.
Technical Differentiation
- Reliable condition monitoring and fault diagnosis through a distinctive 2-stage alarm system
- Existing industrial complexes have been operated for over 20 years and are in urgent need of safety management. Through
systemized risk management, an active risk management technology can be applied to allow highly efficient, low-cost
maintenance and life extension, which can be expanded to related fields.
- From the long-term aspect, diagnosis and condition-based maintenance (CBM) technology is needed to guarantee the system
soundness and reliability.
- Apply Algorithm and signal system that fulfills international standards (DNV·GL, IEC 61400-25)
Excellence of Technology
- Fault diagnosis and prognosis algorithms verified by using the wind power generation simulator
- Accuracy of fault diagnosis over 99%, RUL prediction error below 10%
- The fault diagnosis and RUL prediction for main components can be effectively continued during the varying load due to the wind
(characteristics of wind turbine operation), and the applicability and performance of this technology has been confirmed with the
data from an actual wind turbine.
- Acquirement of condition monitoring system (CMS) and monitoring body certifications from KR and GL.
- CMS installed and operated by 17 units in Complex 1 and 2 at Yeongheung wind power plant of Korea South-East Power Co., Ltd.
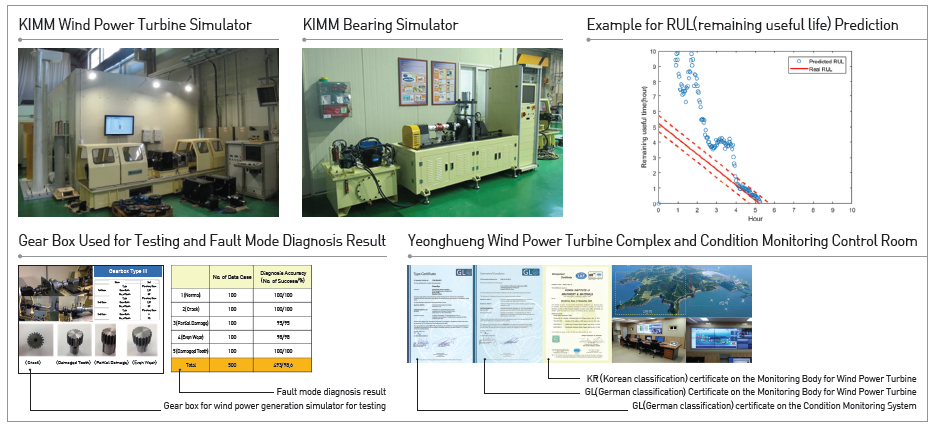
CURRENT INTELLECTUAL PROPERTY RIGHT STATUS
PATENT
- Condition Monitoring Apparatus and Condition Monitoring Method for Machinery System (KR1166871)
- Fault Diagnosis of Wind Turbine by Using Active Bin (KR1420846)
- Decimation Method for Measurement Data of Wind Turbine Condition Monitoring System Using Active Bins (KR1398072)
- A Method of Setting Alarm Levels for Condition Monitoring and Fault Diagnosis of Wind Turbine Generator (KR1599210)
- A System and Method for Monitoring Offshore Wind Turbine Structure (KR1740896)
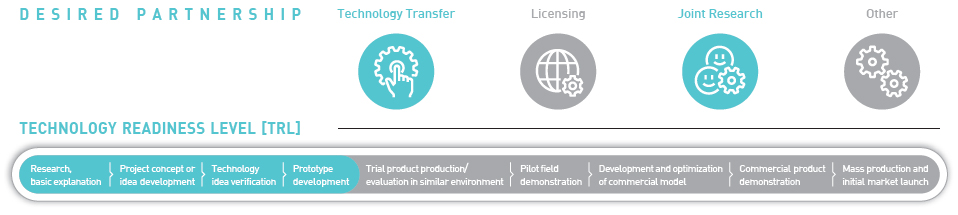
|